Modeling COVID-19 Confirmed Cases Using a Hybrid Model
DOI:
https://doi.org/10.47654/v26y2022i1p128-162Abstract
Purpose: The COVID-19 virus has caused numerous problems worldwide. Given the negative effects of COVID-19, this study aims to estimate accurate forecasts of the number of confirmed cases to help policymakers determine and make the right decisions.
Design/methodology/approach: This paper uses a hybrid approach for forecasting the daily COVID-19 cases based on combining the Autoregressive Integrated Moving Average (ARIMA) and Autoregressive Neural Network (NNAR) with a single hidden layer. To fit the linear pattern from the data, ARIMA models are used. Then, the NNAR models are used to capture the nonlinear pattern. The final prediction is obtained by adding up the two predictions.
Findings: Using six-time series from January 22, 2020, to June 22, 2021, of new daily confirmed cases of COVID-19 from Pakistan, Tunisia, Indonesia, Malaysia, India and South Korea, this work evaluates the hybrid approach against some benchmark models and generated ten days ahead forecasts. Experiments demonstrate the superiority of the hybrid model over the benchmark models.
Originality/value: Given the complex nature of new confirmed cases, it is assumed that the data contains both linear and nonlinear components. In literature, different studies have tended to forecast future cases of COVID-19. However, most of them have used single models that capture either linear or nonlinear patterns. This paper proposes a hybrid model that captures both linear and nonlinear components from the data.
References
Abbasimehr, H., Paki, R., & Bahrini, A. (2021). A novel approach based on combining deep learning models with statistical methods for COVID-19 time series forecasting. Neural Computing and Applications, 34(4), 3135–3149.https://doi.org/10.1007/s00521-021-06548-9
Adhikari, R., & Agrawal, R. K. (2013). A combination of artificial neural network and random walk models for financial time series forecasting. Neural Computing and Applications, 24(6), 1441–1449. https://doi.org/10.1007/s00521-013-1386-y
Agyei, S. K., Isshaq, Z., Frimpong, S., Adam, A. M., Bossman, A., & Asiamah, O. (2021). COVID‐19 and food prices in sub‐Saharan Africa. African Development Review, 33(S1).https://doi.org/10.1111/1467-8268.12525
Anderson, O. D., Granger, C. W. J., & Andersen, A. P. (1979).An Introduction to Bilinear Time Series Models.Applied Statistics, 28(3), 305.https://doi.org/10.2307/2347208
Aras, S., & Kocakoç, P. D. (2016).A new model selection strategy in time series forecasting with artificial neural networks: IHTS. Neurocomputing, 174, 974–987. https://doi.org/10.1016/j.neucom.2015.10.036
Babu, C. N., & Reddy, B. E. (2014). A moving-average filter based hybrid ARIMA–ANN model for forecasting time series data. Applied Soft Computing, 23, 27–38.https://doi.org/10.1016/j.asoc.2014.05.028
Büyükşahin, M. A., & Ertekin, E. (2019).Improving forecasting accuracy of time series data using a new ARIMA-ANN hybrid method and empirical mode decomposition.Neurocomputing, 361, 151–163. https://doi.org/10.1016/j.neucom.2019.05.099
Callen, J. L., Kwan, C. C., Yip, P. C., & Yuan, Y. (1996).Neural network forecasting of quarterly accounting earnings. International Journal of Forecasting, 12(4), 475–482. https://doi.org/10.1016/s0169-2070(96)00706-6
Casey Brace, M., Schmidt, J., & Hadlin, M. (1991). Comparison of the forecasting accuracy of neural networks with other established techniques. Proceedings of the First International Forum on Applications of Neural Networks to Power Systems. https://doi.org/10.1109/ann.1991.213493
Chakraborty, T., & Ghosh, I. (2020). Real-time forecasts and risk assessment of novel coronavirus (COVID-19) cases: A data-driven analysis. Chaos, Solitons& Fractals, 135, 109850. https://doi.org/10.1016/j.chaos.2020.109850
Clemen, R. T. (1989). Combining forecasts: A review and annotated bibliography. International Journal of Forecasting, 5(4), 559–583. https://doi.org/10.1016/0169-2070(89)90012-5
Denton, J. W. (1995), "How good are neural networks for causal forecasting?"The Journal of Business Forecasting, 14, 17.
Engle, R. F. (1982). Autoregressive Conditional Heteroscedasticity with Estimates of the Variance of United Kingdom Inflation.Econometrica, 50(4), 987.https://doi.org/10.2307/1912773
Foster, W., Collopy, F., & Ungar, L. (1992). Neural network forecasting of short, noisy time series.Computers & Chemical Engineering, 16(4), 293–297. https://doi.org/10.1016/0098-1354(92)80049-f
Hann, T. H., & Steurer, E. (1996).Much ado about nothing? Exchange rate forecasting: Neural networks vs. linear models using monthly and weekly data. Neurocomputing, 10(4), 323–339. https://doi.org/10.1016/0925-2312(95)00137-9
Huang, C., Wang, Y., Li, X., Ren, L., Zhao, J., Hu, Y., Zhang, L., Fan, G., Xu, J., Gu, X., Cheng, Z., Yu, T., Xia, J., Wei, Y., Wu, W., Xie, X., Yin, W., Li, H., Liu, M., . . . Cao, B. (2020). Clinical features of patients infected with 2019 novel coronavirus in Wuhan, China. The Lancet, 395(10223), 497–506. https://doi.org/10.1016/s0140-6736(20)30183-5
Hyndman, R. J., & Athanasopoulos, G. (2021). Forecasting: Principles and Practice (3rd ed.). Otexts.
Hyndman, R. J., &Khandakar, Y. (2008). Automatic Time Series Forecasting: The forecast Package for R. Journal of Statistical Software, 27(3).https://doi.org/10.18637/jss.v027.i03
Khashei, M., & Bijari, M. (2011).A novel hybridization of artificial neural networks and ARIMA models for time series forecasting.Applied Soft Computing, 11(2), 2664–2675.https://doi.org/10.1016/j.asoc.2010.10.015
Kırbaş, S., Sözen, A., Tuncer, A. D., & Kazancıoğlu, F. I. (2020).Comparative analysis and forecasting of COVID-19 cases in various European countries with ARIMA, NARNN and LSTM approaches. Chaos, Solitons& Fractals, 138, 110015.https://doi.org/10.1016/j.chaos.2020.110015
Moftakhar, L., Seif, M., & Safe, M. S. (2020). Exponentially Increasing Trend of Infected Patients with COVID-19 in Iran: A Comparison of Neural Network and ARIMA Forecasting Models. Iranian Journal of Public Health. https://doi.org/10.18502/ijph.v49is1.3675
Moslehpour, M., Al-Fadly, A., Ehsanullah, S. et al. (2022). Assessing Financial Risk Spillover and Panic Impact of Covid-19 on European and Vietnam Stock market. Environ Sci Pollut Res. https://doi.org/10.1007/s11356-021-18170-2
Ömer Faruk, D. (2010). A hybrid neural network and ARIMA model for water quality time series prediction. Engineering Applications of Artificial Intelligence, 23(4), 586–594 .https://doi.org/10.1016/j.engappai.2009.09.015
Owusu Junior, P., Frimpong, S., Adam, A. M., Agyei, S. K., Gyamfi, E. N., Agyapong, D., & Tweneboah, G. (2021). COVID-19 as Information Transmitter to Global Equity Markets: Evidence from CEEMDAN-Based Transfer Entropy Approach. Mathematical Problems in Engineering, 2021, 1–19. https://doi.org/10.1155/2021/8258778
Ravazzolo, F., Casarin, R., Corradin, F., & Sartore, D. (2020). A scoring rule for factor and autoregressive models under misspecification. Advances in Decision Sciences. Asia University, Taiwan. 24(2), 66-103. https://doi.org/10.47654/v24y2020i2p66-103
Safi, S. K., Sanusi, O. I., & Tabash, M. I. (2022). Forecasting the Impact of COVID-19 Epidemic on China Exports using Different Time Series Models. Advances in Decision Sciences. Asia University, Taiwan. 26(1), 102-127. https://doi.org/10.47654/v26y2022i1p102-127
Tajmouati, S., Wahbi, B. E., Bedoui, A., Abarda, A., & Dakkoun, M. (2021). Applying k-nearest neighbors to time series forecasting: two new approaches. arXiv preprint arXiv:2103.14200. https://arxiv.org/abs/2103.14200
Tajmouati, S., Wahbi, B. W. B., Bedoui, A., ABARDA, A., & Dakkoun, M. (2020).Une nouvelle approche d’application de l’algorithme K-NN pour la prévision du nombre total des cas confirmés de COVID-19. Journal of Integrated Studies In Economics, Law, Technical Sciences & Communication, 2(1). https://revues.imist.ma/index.php/JISELSC/article/view/24760
Tong, H. (2011). Threshold models in time series analysis — 30 years on. Statistics and Its Interface, 4(2), 107–118. https://doi.org/10.4310/sii.2011.v4.n2.a1
Torrealba-Rodriguez, O., Conde-Gutiérrez, R., & Hernández-Javier, A. (2020). Modeling and prediction of COVID-19 in Mexico applying mathematical and computational models. Chaos, Solitons & Fractals, 138, 109946.https://doi.org/10.1016/j.chaos.2020.109946
Tran, T., Pham, L., and Ngo, Q. (2020), "Forecasting epidemic spread of SARS-CoV-2 using ARIMA model (Case study: Iran),"Global Journal of Environmental Science and Management, 6, 1–10. 10.22034/GJESM.2019.06.SI.01
Zhang, G. (2003). Time series forecasting using a hybrid ARIMA and neural network model. Neurocomputing, 50, 159–175. https://doi.org/10.1016/s0925-2312(01)00702-0
Ziegel, E. R., Box, G., Jenkins, G., & Reinsel, G. (1995). Time Series Analysis, Forecasting, and Control. Technometrics, 37(2), 238.https://doi.org/10.2307/1269640
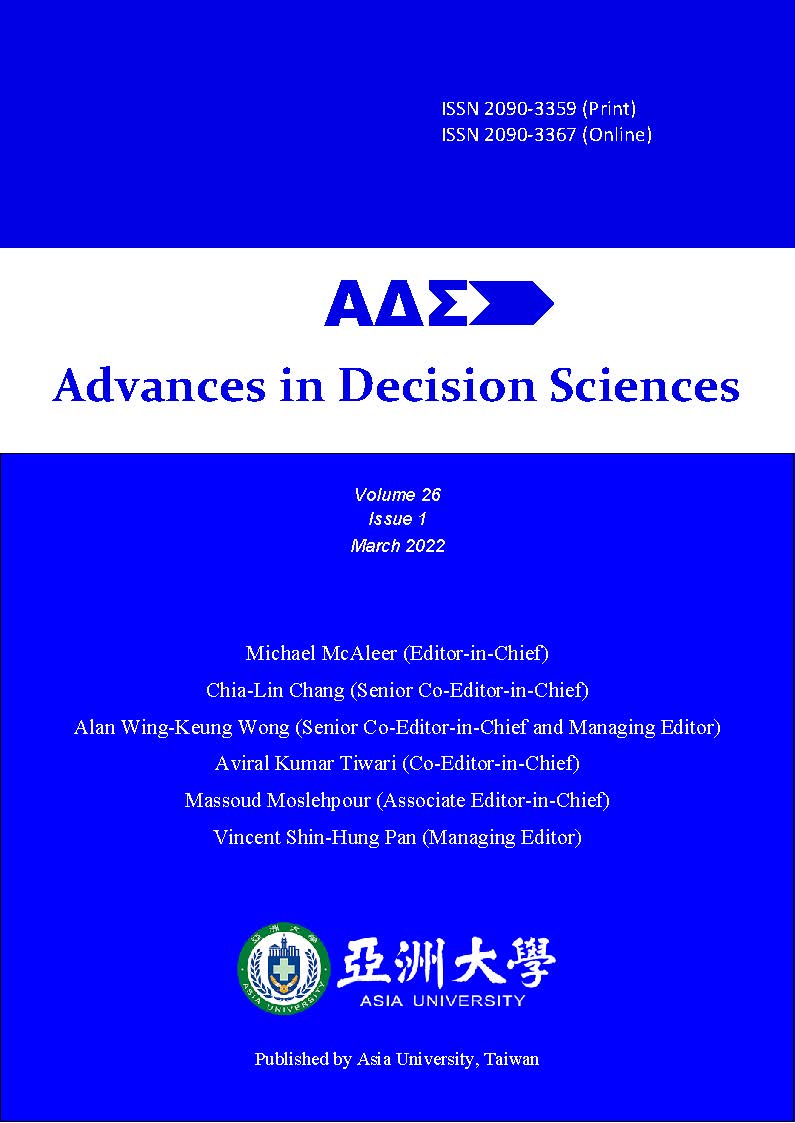
Published
Issue
Section
License
Copyright (c) 2021 Advances in Decision Sciences

This work is licensed under a Creative Commons Attribution-NonCommercial 4.0 International License.