A Decision Science Approach Using Hybrid EEG Feature Extraction and GAN-Based Emotion Classification
DOI:
https://doi.org/10.47654/v27y2023i1p172-191Keywords:
EEG, Emotions, DEAP Data, GAN, Independent Component AnalysisAbstract
Purpose: Emotions play an essential role in human life and they profoundly influence behavior, decision-making, and well-being. This approach aims to classify human emotions by using Generative Adversarial Networks (GAN) and hybrid Electroencephalography (EEG) features with the DEAP dataset.
Design/methodology/approach: The proposed system addresses the limitations of traditional classification techniques by generating synthetic hybrid features that capture additional information about emotional states. Informed by decision science principles, the system recognizes that emotions heavily influence human decision-making processes.
Findings: The process consists of data collection, pre-processing, feature extraction, GAN training, hybrid feature generation, and classification. The DEAP dataset is pre-processed by using Independent Component Analysis (ICA) and Wavelet Transform to remove artifacts. A GAN model is trained to generate synthetic features that mimic the distribution of real EEG signals. The hybrid features are generated by combining the real EEG features and synthetic features.
Originality/value: The performance of the classification system is evaluated using accuracy at 97.4%, precision at 97.22%, recall at 96.8%, and F1 score at 97.08%. By leveraging EEG signals, the proposed system shows promise in enhancing the accuracy of emotion classification, opening up exciting avenues for future research in this domain.
References
Acevedo, R. A., Aponte, E., Harmath, P., & Mora Mora, J. (2021). Rational irrationality: A two stage decision making model. Journal of Advances in Decision Science, 25(1).
Ashokkumar, S. R., Anupallavi, S., MohanBabu, G., Premkumar, M., & Jeevanantham, V. (2022). Emotion identification by dynamic entropy and ensemble learning from electroencephalogram signals. International Journal of Imaging Systems and Technology, 32(1), 402-413.
Ashokkumar, S. R., Premkumar, M., Anupallavi, S., Jeevanantham, V., Mohanbabu, G., & Selvapandian, A. (2023). Quantifying Functional Connectivity Network Through Synchronization and Graph Theory Approaches for Seizure Prediction. Wireless Personal Communications, 129(1), 747-780
Batmunkh, M. U., Choijil, E., Vieito, J. P., Espinosa-Méndez, C., & Wong, W. K. (2020). Does herding behavior exist in the Mongolian stock market?. Pacific-Basin Finance Journal, 62, 101352.
Chen, J., Jiang, D., Zhang, Y., & Zhang, P. (2020). Emotion recognition from spatiotemporal EEG representations with hybrid convolutional recurrent neural networks via wearable multi-channel headset. Computer Communications, 154, 58-65.
Cheng, J., Chen, M., Li, C., Liu, Y., Song, R., Liu, A., & Chen, X. (2020). Emotion recognition from multi-channel EEG via deep forest. IEEE Journal of Biomedical and Health Informatics, 25(2), 453-464.
Dhanasekaran, S., Gomathi, P., Maximus, A. R., Krishnan, T., & Kannan, B. (2022, December). Solar Tree based Smart City Street Light Control System using IoT BLYNK Platform. In 2022 International Conference on Automation, Computing and Renewable Systems (ICACRS) (pp. 284-290). IEEE.
Dhanasekaran, S., Mathiyalagan, P., & Rajeshwaran, A. M. (2021). Automatic Segmentation of Lung Tumors Using Adaptive Neuron-Fuzzy Inference System. Annals of the Romanian Society for Cell Biology, 17468-17483.
Fong, W. M., Lean, H. H., & Wong, W. K. (2008). Stochastic dominance and behavior towards risk: The market for internet stocks. Journal of Economic Behavior & Organization, 68(1), 194-208.
Gao, Y., Fu, X., Ouyang, T., & Wang, Y. (2022). EEG-GCN: spatio-temporal and self-adaptive graph convolutional networks for single and multi-view EEG-based emotion recognition. IEEE Signal Processing Letters, 29, 1574-1578.
Gohar, R., Bagadeem, S., Chang, B. H., & Zong, M. (2022). Do the income and price changes affect consumption in the emerging 7 countries? Empirical evidence using quantile ARDL model. Annals of Financial Economics, 17(04), 2250024.
Jebril, I., Dhanaraj, P., Abdulsahib, G. M., Palanisamy, S., Prabhu, T., & Khalaf, O. I. (2022). Analysis of Electrically Couple SRR EBG Structure for Sub 6 GHz Wireless Applications. Advances in Decision Sciences, 26(5), 102-123. 102-123.
Kalna-Dubinyuk, T., Ladychenko, K. I., Syerova, L. P., Kuchma, M., & Litovka-Demenina, S. G. (2023). Sequential analysis of variants as a new method of dynamic modeling in making scientifically grounded business decisions. Advances in Decision Sciences, 27(1), 1-22.
Karatas, C., & Unal, G. (2022). Causality, Information Flow, And Co-Movement Analysis of Major Stock Indices. Annals of Financial Economics, 17(03), 2250019.
Kilic, E., and Göksel, O. (2021). Arbitrageur Behavior In Sentiment-Driven Asset-Pricing. Annals of Financial Economics, 16(03), 2150015.
Koelstra, S., Muhl, C., Soleymani, M., Lee, J. S., Yazdani, A., Ebrahimi, T., Pun, T., Nijholt, A., & Patras, I. (2012). DEAP: A database for emotion analysis using physiological signals. IEEE Transactions on Affective Computing, 3(1), 18-31. https://doi.org/10.1109/T-AFFC.2011.15
Kudryavtsev, A. (2019). Short-term herding effect on market index returns. Annals of Financial Economics, 14(01), 1950004.
Lam, K., Liu, T., & Wong, W. K. (2012). A new pseudo-Bayesian model with implications for financial anomalies and investors’ behavior. Journal of Behavioral Finance, 13(2), 93-107.
Li, P., Liu, H., Si, Y., Li, C., Li, F., Zhu, X., ... & Xu, P. (2019). EEG based emotion recognition by combining functional connectivity network and local activations. IEEE Transactions on Biomedical Engineering, 66(10), 2869-2881.
Li, Z., Jiang, H., Chen, Z., & Wong, W. K. (2022). A mental account-based portfolio selection model with an application for data with smaller dimensions. Computers & Operations Research, 144, 105801.
Lian, J., Zhang, Y., Luo, R., Han, G., Jia, W., & Li, C. (2020). Pair-wise matching of eeg signals for epileptic identification via convolutional neural network. IEEE Access, 8, 40008-40017.
Liu, H., Zhang, Y., Li, Y., & Kong, X. (2021). Review on Emotion Recognition Based on Electroencephalography. Frontiers in computational neuroscience, 15, 758212. https://doi.org/10.3389/fncom.2021.758212
Liu, Y. C., Chen, H. J., & Hsu, W. T. (2020). Prior Trading Outcomes And Subsequent Portfolio Risks: Using Securities Dealer In Taiwan As An Example. Annals of Financial Economics, 15(03), 2050012.
Luo, Y., & Lu, B. L. (2018, July). EEG data augmentation for emotion recognition using a conditional Wasserstein GAN. In 2018 40th annual international conference of the IEEE engineering in medicine and biology society (EMBC) (pp. 2535-2538). IEEE.
Mandala, V., Senthilnathan, T., Suganyadevi, S., Gobhinat, S., Selvaraj, D., & Dhanapal, R. (2023). An optimized back propagation neural network for automated evaluation of health condition using sensor data. Measurement: Sensors, 100846.
Mangalampalli, S., Karri, G. R., Kumar, M., Khalaf, O. I., Romero, C. a. T., & Sahib, G. A. (2023). DRLBTSA: Deep reinforcement learning based task-scheduling algorithm in cloud computing. Multimedia Tools and Applications. https://doi.org/10.1007/s11042-023-16008-2
Pandey, P., & Seeja, K. R. (2021). Subject independent emotion recognition system for people with facial deformity: an EEG based approach. Journal of Ambient Intelligence and Humanized Computing, 12, 2311-2320. https://doi.org/10.1007/s12652-020-02338-8
Rufus, N. H. A., Anand, D., Rama, R. S., Kumar, A., & Vigneshwar, A. S. (2022, November). Evolutionary Optimization with Deep Transfer Learning for Content based Image Retrieval in Cloud Environment. In 2022 International Conference on Augmented Intelligence and Sustainable Systems (ICAISS) (pp. 826-831). IEEE.
Topic, A., & Russo, M. (2021). Emotion recognition based on EEG feature maps through deep learning network. Engineering Science and Technology, an International Journal, 24(6), 1442-1454.
Vandarkuzhali, J., Kamatchi, S., Chakravarthi, M. K., Selvaraj, D., & Dhanapal, R. (2023). Hybrid RF and PCA method: The number and Posture of piezoresistive sensors in a multifunctional technology for respiratory monitoring. Measurement: Sensors, 100832.
Xue, X., Chinnaperumal, S., Abdulsahib, G. M., Manyam, R. R., Marappan, R., Raju, S. K., & Khalaf, O. I. (2023). Design and Analysis of a Deep Learning Ensemble Framework Model for the Detection of COVID-19 and Pneumonia Using Large-Scale CT Scan and X-ray Image Datasets. Bioengineering, 10(3), 363.
Xue, X., Shanmugam, R., Palanisamy, S., Khalaf, O. I., Selvaraj, D., & Abdulsahib, G. M. (2023). A hybrid cross layer with harris-hawk-optimization-based efficient routing for wireless sensor networks. Symmetry, 15(2), 438.
Zhang, Y., Chen, J., Tan, J. H., Chen, Y., Chen, Y., Li, D., ... & Che, W. (2020). An investigation of deep learning models for EEG-based emotion recognition. Frontiers in Neuroscience, 14, 622759.
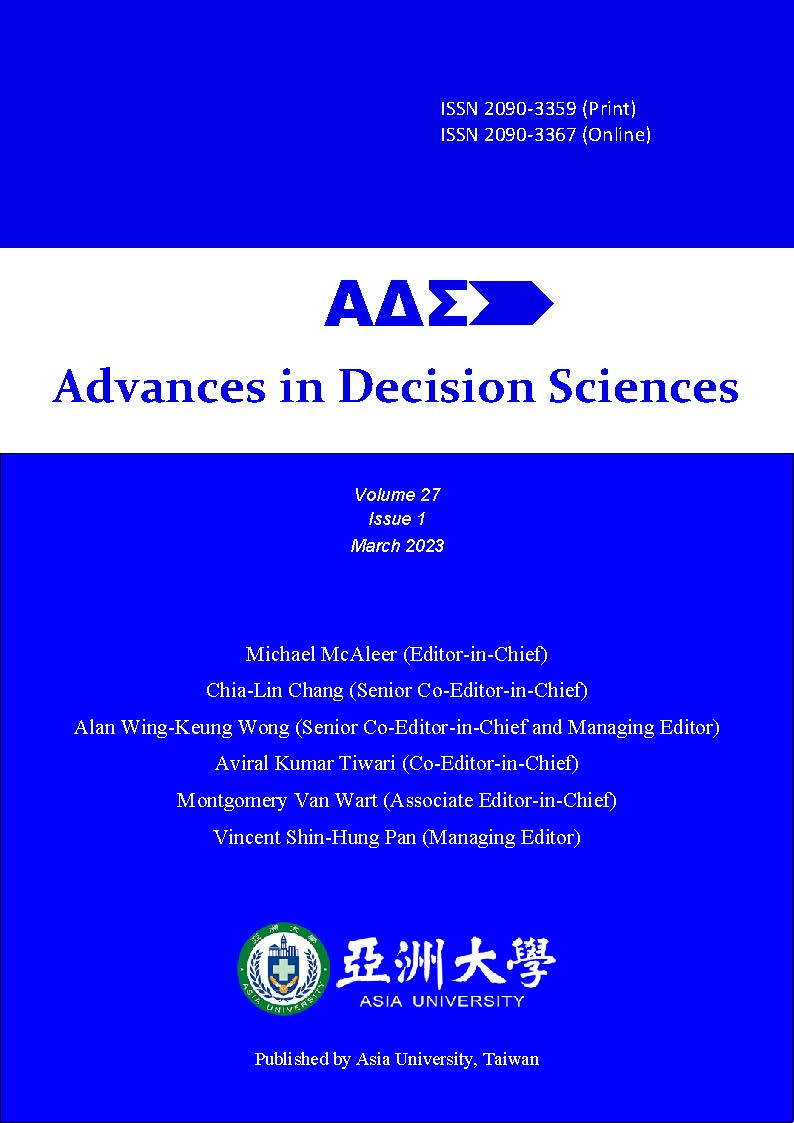
Published
Issue
Section
License
Copyright (c) 2023 Advances in Decision Sciences

This work is licensed under a Creative Commons Attribution-NonCommercial 4.0 International License.