Investigating the effects of Accounting Law on the Credit Rating Models using Artificial Neural Networks: a study in Vietnam
DOI:
https://doi.org/10.47654/v26y2022i4p17-49Keywords:
local accounting law, Vietnamese accounting system, credit classification, credit rating, Artificial Intelligence (AI), Artificial Neural Networks (ANN), firms in VietnamAbstract
Purpose: This study builds up a credit rating model for small, medium, and large firms in Vietnam in the period from 2008 to 2018 and applies the model to analyse the effect of the new accounting law on credit rating. This research has several contributions to the literature. First, this study investigates the local accounting law which significantly affects the credit rating for small, medium, and large firms in Vietnam by using Artificial Neural Networks (ANN). To illustrate, the new accounting law changes the measures in the financial reports of firms, including assets, liabilities, owner’s equity, revenues, and expenses. Thus, it will affect the credit rating.
Design/ Methodology/ Approach: In this research, the dataset includes 39,162 small, medium, and large firms in Vietnam in the period 2008–2018 from the Orbis Database using Artificial Neural Networks (ANN). For the first time in the literature, this research analyses the effect of the new accounting law on credit rating for Vietnamese small, medium, and large firms by using ANN.
Findings: The result of this study shows that the new local accounting system significantly affects the credit rating in several ways: (1) by changing the inputs of the models, (2) by changing the model performance, and (3) by changing the exact values of the weights and biases of the models. This research also finds evidence that the ANN model for the period from 2008 to 2014 (before implementing Circular 200) has a better predicting power than that for the period from 2015 to 2018 (after implementing Circular 200).
Practical implication: This study investigates the new accounting system (Circular 200) implemented on 1 January 2015 in Vietnam which guides both local and foreign enterprises in accounting policies for financial years beginning 1 January 2015.
References
Adebayo, T. S., Kirikkaleli, D., & Rjoub, H. (2022). Time–frequency analysis between economic risk and financial risk in the mint nations: What causes what? Annals of Financial Economics, 17(02), 2250013. https://doi.org/10.1142/S2010495222500130
Affes, H., & Callimaci, A. (2007). Les déterminants de l’adoption anticipée des normes comptables internationales: Choix financier ou opportunisme? Comptabilité-Contrôle-Audit, 13(2), 149–166.
Altman, E. I. (1968). Financial Ratios, Discriminant Analysis And The Prediction Of Corporate Bankruptcy. The Journal of Finance, 23(4), 589–609. https://doi.org/10.1111/j.1540-6261.1968.tb00843.x
Altman, E. I., Iwanicz‐Drozdowska, M., Laitinen, E. K., & Suvas, A. (2017). Financial distress prediction in an international context: A review and empirical analysis of Altman’s Z‐score model. Journal of International Financial Management & Accounting, 28(2), 131–171.
Altman, E. I., & Sabato, G. (2007). Modelling Credit Risk for SMEs: Evidence from the U.S. Market. Abacus, 43(3), 332–357. https://doi.org/10.1111/j.1467-6281.2007.00234.x
Angelini, E., di Tollo, G., & Roli, A. (2008). A neural network approach for credit risk evaluation. The Quarterly Review of Economics and Finance, 48(4), 733–755. https://doi.org/10.1016/j.qref.2007.04.001
Atiya, A. F. (2001). Bankruptcy prediction for credit risk using neural networks: A survey and new results. IEEE Transactions on Neural Networks, 12(4), 929–935. https://doi.org/10.1109/72.935101
Aye, G. C., & Odhiambo, N. M. (2021). Threshold effect of inflation on agricultural growth: Evidence from developing countries. Advances in Decision Sciences, 25(2), 1–22.
Barth, M. E. (1994). Fair value accounting: Evidence from investment securities and the market valuation of banks. Accounting Review, 1–25.
Barth, M. E., Beaver, W. H., & Landsman, W. R. (1996). Value-relevance of banks’ fair value disclosures under SFAS No. 107. Accounting Review, 513–537.
Barth, M. E., & Kasznik, R. (1999). Revaluations of fixed assets and future firm performance: Evidence from the UK. Journal of Accounting and Economics, 26(1–3), 149–178.
Barth, M. E., Landsman, W. R., & Lang, M. H. (2008). International accounting standards and accounting quality. Journal of Accounting Research, 46(3), 467–498.
Bartov, E., Goldberg, S. R., & Kim, M. (2005). Comparative value relevance among German, US, and international accounting standards: A German stock market perspective. Journal of Accounting, Auditing & Finance, 20(2), 95–119.
Benmelech, E., & Dlugosz, J. (2009). The Credit Rating Crisis. NBER Macroeconomics Annual. https://doi.org/10.1086/648293
Benston, G. J. (2006). Fair-value accounting: A cautionary tale from Enron. Journal of Accounting and Public Policy, 25(4), 465–484.
Blanchet, F. G., Legendre, P., & Borcard, D. (2008). Forward selection of explanatory variables. Ecology, 89(9), 2623–2632.
Campbell, J. Y., Hilscher, J., & Szilagyi, J. (2008). In Search of Distress Risk. The Journal of Finance, 63(6), 2899–2939. https://doi.org/10.1111/j.1540-6261.2008.01416.x
Carmona, S., & Trombetta, M. (2008). On the global acceptance of IAS/IFRS accounting standards: The logic and implications of the principles-based system. Journal of Accounting and Public Policy, 27(6), 455–461.
Chai, N., Wu, B., Yang, W., & Shi, B. (2019). A multicriteria approach for modeling small enterprise credit rating: Evidence from China. Emerging Markets Finance and Trade, 55(11), 2523–2543.
Cornée, S. (2019). The Relevance of Soft Information for Predicting Small Business Credit Default: Evidence from a Social Bank. Journal of Small Business Management, 57(3), 699–719. https://doi.org/10.1111/jsbm.12318
Cuijpers, R., & Buijink, W. (2005). Voluntary adoption of non-local GAAP in the European Union: A study of determinants and consequences. European Accounting Review, 14(3), 487–524.
Daske, H., & Gebhardt, G. (2006). International financial reporting standards and experts’ perceptions of disclosure quality. Abacus, 42(3‐4), 461–498.
Dias, R. (2013). The Rise and Fall of Credit Default Swaps: An empirical investigation of global banks and non-bank financial institutions [Ph.D.]. Swinburne University of Technology.
Ding, Y., Hope, O.-K., Jeanjean, T., & Stolowy, H. (2007). Differences between domestic accounting standards and IAS: Measurement, determinants and implications. Journal of Accounting and Public Policy, 26(1), 1–38.
Doumpos, M., Lemonakis, C., Niklis, D., & Zopounidis, C. (2019). Analytical Techniques in the Assessment of Credit Risk: An Overview of Methodologies and Applications. Springer International Publishing. https://doi.org/10.1007/978-3-319-99411-6
Duan, J.-C., Sun, J., & Wang, T. (2012). Multiperiod corporate default prediction—A forward intensity approach. Journal of Econometrics, 170(1), 191–209. https://doi.org/10.1016/j.jeconom.2012.05.002
Dumontier, P., & Raffournier, B. (1998). Why firms comply voluntarily with IAS: an empirical analysis with Swiss data. Journal of International Financial Management & Accounting, 9(3), 216–245.
El-Gazzar, S. M., Finn, P. M., & Jacob, R. (1999). An empirical investigation of multinational firms’ compliance with international accounting standards. The International Journal of Accounting, 34(2), 239–248.
Farhadieh, F. (2011). A Statistical Framework for Quantifying Adaptive Behavioural Risk for the Banking Industry [Ph.D.]. Swinburne University of Technology.
Florou, A., Kosi, U., & Pope, P. F. (2017). Are international accounting standards more credit relevant than domestic standards? Accounting and Business Research, 47(1), 1–29. https://doi.org/10.1080/00014788.2016.1224968
Giordani, P., Jacobson, T., Schedvin, E. von, & Villani, M. (2014). Taking the Twists into Account: Predicting Firm Bankruptcy Risk with Splines of Financial Ratios. Journal of Financial and Quantitative Analysis, 49(4), 1071–1099. https://doi.org/10.1017/S0022109014000623
Goonatilake, S., & Treleaven, P. C. (1995). Intelligent systems for finance and business. John Wiley & Sons, Inc.
Hamori, S., & Kume, T. (2018). Artificial intelligence and economic growth. Advances in Decision Sciences, 22, 1–22.
Hao, N. T. N., & Wong, W.-K. (2021). Does Bank Liquidity Risk Lead to Bank’s Operational Efficiency? A Study in Vietnam. Advances in Decision Sciences, 25(4), 1–43.
Hodder, L., Hopkins, P., & Schipper, K. (2014). Fair value measurement in financial reporting. Foundations and Trends® in Accounting, 8(3–4), 143–270.
Hope, O.-K., Jin, J., & Kang, T. (2006). Empirical evidence on jurisdictions that adopt IFRS. Journal of International Accounting Research, 5(2), 1–20.
Jacobson, T., Lindé, J., & Roszbach, K. (2013). Firm Default and Aggregate Fluctuations. Journal of the European Economic Association, 11(4), 945–972. https://doi.org/10.1111/jeea.12020
Jones, S., & Higgins, A. D. (2006). Australia’s switch to international financial reporting standards: A perspective from account preparers. Accounting & Finance, 46(4), 629–652.
Jones, S., Johnstone, D., & Wilson, R. (2017). Predicting Corporate Bankruptcy: An Evaluation of Alternative Statistical Frameworks: AN EVALUATION OF ALTERNATIVE STATISTICAL FRAMEWORKS. Journal of Business Finance & Accounting, 44(1–2), 3–34. https://doi.org/10.1111/jbfa.12218
Kumar, K., & Bhattacharya, S. (2006). Artificial neural network vs linear discriminant analysis in credit ratings forecast. Review of Accounting and Finance. https://doi.org/10.1108/14757700610686426
Langkamp, D. L., Lehman, A., & Lemeshow, S. (2010). Techniques for Handling Missing Data in Secondary Analyses of Large Surveys. Academic Pediatrics, 10(3), 205–210. https://doi.org/10.1016/j.acap.2010.01.005
Lantto, A.-M., & Sahlström, P. (2009). Impact of International Financial Reporting Standard adoption on key financial ratios. Accounting & Finance, 49(2), 341–361. https://doi.org/10.1111/j.1467-629X.2008.00283.x
Laux, C., & Leuz, C. (2009). The crisis of fair-value accounting: Making sense of the recent debate. Accounting, Organizations and Society, 34(6–7), 826–834.
Le, B. T. (2018). Factors affecting credit risk management system of the Vietnamese commercial banks [University of Economics Ho Chi Minh City]. http://digital.lib.ueh.edu.vn/handle/UEH/57976
Leuz, C., & Verrecchia, R. E. (2000). The economic consequences of increased disclosure. Journal of Accounting Research, 91–124.
Lok, C.-L., Chuah, S.-F., & Hooy, C.-W. (2022). The Impacts Of Data-Driven Leadership In Ir4. 0 Adoption On Firm Performance In Malaysia. Annals of Financial Economics, 17(03), 2250023.
Mahmood, F., Shahzad, U., Nazakat, A., Ahmed, Z., Rjoub, H., & Wong, W.-K. (2022). The nexus between cash conversion cycle, working capital finance, and firm performance: Evidence from novel machine learning approaches. Annals of Financial Economics, 17(02), 2250014. https://doi.org/10.1142/S2010495222500142
Mankiw, N. G. (2020). Brief principles of macroeconomics. Cengage Learning.
Matlab. (2019a). Analyze Shallow Neural Network Performance After Training—MATLAB & Simulink. https://www.mathworks.com/help/deeplearning/ug/analyze-neural-network-performance-after-training.html
Matlab. (2019b). Bayesian regularization backpropagation—MATLAB trainbr—MathWorks Australia. https://au.mathworks.com/help/deeplearning/ref/trainbr.html;jsessionid=13db9f22dcc3a877e7628befef6d
Matlab. (2019c). Train and Apply Multilayer Shallow Neural Networks—MATLAB & Simulink. https://www.mathworks.com/help/deeplearning/ug/train-and-apply-multilayer-neural-networks.html
ModeFinance. (2017). The Multi Objective Rating Evaluation Model (MORE). https://cra.modefinance.com/pdf/modefinanceCorporatesScoringMethodology.pdf
Morales-Díaz, J., & Zamora-Ramírez, C. (2018). The Impact of IFRS 16 on Key Financial Ratios: A New Methodological Approach. Accounting in Europe, 15(1), 105–133. https://doi.org/10.1080/17449480.2018.1433307
Muñoz‐Izquierdo, N., Laitinen, E. K., Camacho‐Miñano, M., & Pascual‐Ezama, D. (2019). Does audit report information improve financial distress prediction over Altman’s traditional Z-Score model? Journal of International Financial Management & Accounting, 0(0). https://doi.org/10.1111/jifm.12110
Murphy, A. B. (1999). Firm characteristics of Swiss companies that utilize International Accounting Standards. The International Journal of Accounting, 34(1), 121–131.
Nguyen, L.-U. (2019). The (un)suitability of fair-value accounting in emerging economies: The case of Vietnam. Journal of Accounting & Organizational Change. https://doi.org/10.1108/JAOC-03-2018-0032
Nhan, D. T. T., Pho, K.-H., Anh, D. T. V., & Mcaleer, M. (2021). Evaluating the efficiency of vietnam banks using data envelopment analysis. Annals of Financial Economics, 16(02), 2150010. https://doi.org/10.1142/S201049522150010X
Ohlson, J. A. (1980). Financial Ratios and the Probabilistic Prediction of Bankruptcy. Journal of Accounting Research, 18(1), 109–131. JSTOR. https://doi.org/10.2307/2490395
Parker, L. E. (2006). Notes on multilayer, feedforward neural networks. CS494/594: Projects in Machine Learning.
Pham, Q. H., Ho, D., & Khandaker, S. (2022). Credit Rating Models for Firms in Vietnam Using Artificial Neural Networks (ANN). In N. Ngoc Thach, V. Kreinovich, D. T. Ha, & N. D. Trung (Eds.), Financial Econometrics: Bayesian Analysis, Quantum Uncertainty, and Related Topics (pp. 799–833). Springer International Publishing. https://doi.org/10.1007/978-3-030-98689-6_52
Pham, Q. H., Truong, T. L. N., & Bui, D. T. P. (2018). An Alternate Internal Credit Rating System for Construction and Timber Industries Using Artificial Neural Network. Econometrics for Financial Applications, 752–791. https://doi.org/10.1007/978-3-319-73150-6_59
Pham, V. N. B., Do, T. T., & Vo, H. D. (2018). Financial distress and bankruptcy prediction: An appropriate model for listed firms in Vietnam. Economic Systems, 42(4), 616–624. https://doi.org/10.1016/j.ecosys.2018.05.002
Phan, D., Joshi, M., & Tran-Nam, B. (2018). The history of accounting standard setting in an emerging transition economy: The case of Vietnam. Accounting History, 23(3), 379–406. https://doi.org/10.1177/1032373217745672
Psillaki, M., Tsolas, I. E., & Margaritis, D. (2010). Evaluation of credit risk based on firm performance. European Journal of Operational Research, 201(3), 873–881. https://doi.org/10.1016/j.ejor.2009.03.032
Safi, S. K., Sanusi, O. I., & Tabash, M. I. (2022). Forecasting the Impact of COVID-19 Epidemic on China Exports using Different Time Series Models. Advances in Decision Sciences, 26(1), 1–25.
Schechtman, R., & Gaglianone, W. P. (2012). Macro stress testing of credit risk focused on the tails. Journal of Financial Stability, 8(3), 174–192.
Sigrist, F., & Hirnschall, C. (2019). Grabit: Gradient tree-boosted Tobit models for default prediction. Journal of Banking & Finance, 102, 177–192. https://doi.org/10.1016/j.jbankfin.2019.03.004
TajMazinani, M., Hassani, H., & Raei, R. (2022). A Comprehensive Review of Stock Price Prediction Using Text Mining. Advances in Decision Sciences, 26(2), 116–152.
Tran, T., Ha, X., Le, T., & Nguyen, N. (2019). Factors affecting IFRS adoption in listed companies: Evidence from Vietnam. Management Science Letters, 9(13), 2169–2180.
Tsai, M.-C., Lin, S.-P., Cheng, C.-C., & Lin, Y.-P. (2009). The consumer loan default predicting model – An application of DEA–DA and neural network. Expert Systems with Applications, 36(9), 11682–11690. https://doi.org/10.1016/j.eswa.2009.03.009
Wang, F., Sohail, A., Wong, W.-K., Azim, Q. U. A., Farwa, S., & Sajad, M. (2022). Artificial Intelligence and Stochastic Optimization Algorithms for the Chaotic datasets. Fractals. https://doi.org/10.1142/S0218348X22401752
Washington, G. K. (2014). Effects of macroeconomic variables on credit risk in the Kenyan banking system. International Journal of Business and Commerce, 3(9), 1.
Wu, Y., Gaunt, C., & Gray, S. (2010). A comparison of alternative bankruptcy prediction models. Journal of Contemporary Accounting & Economics, 6(1), 34–45. https://doi.org/10.1016/j.jcae.2010.04.002
Xu, R., Wong, W.-K., Chen, G., & Huang, S. (2017). Topological Characteristics of the Hong Kong Stock Market: A Test-based P-threshold Approach to Understanding Network Complexity. Scientific Reports, 7(1), Article 1. https://doi.org/10.1038/srep41379
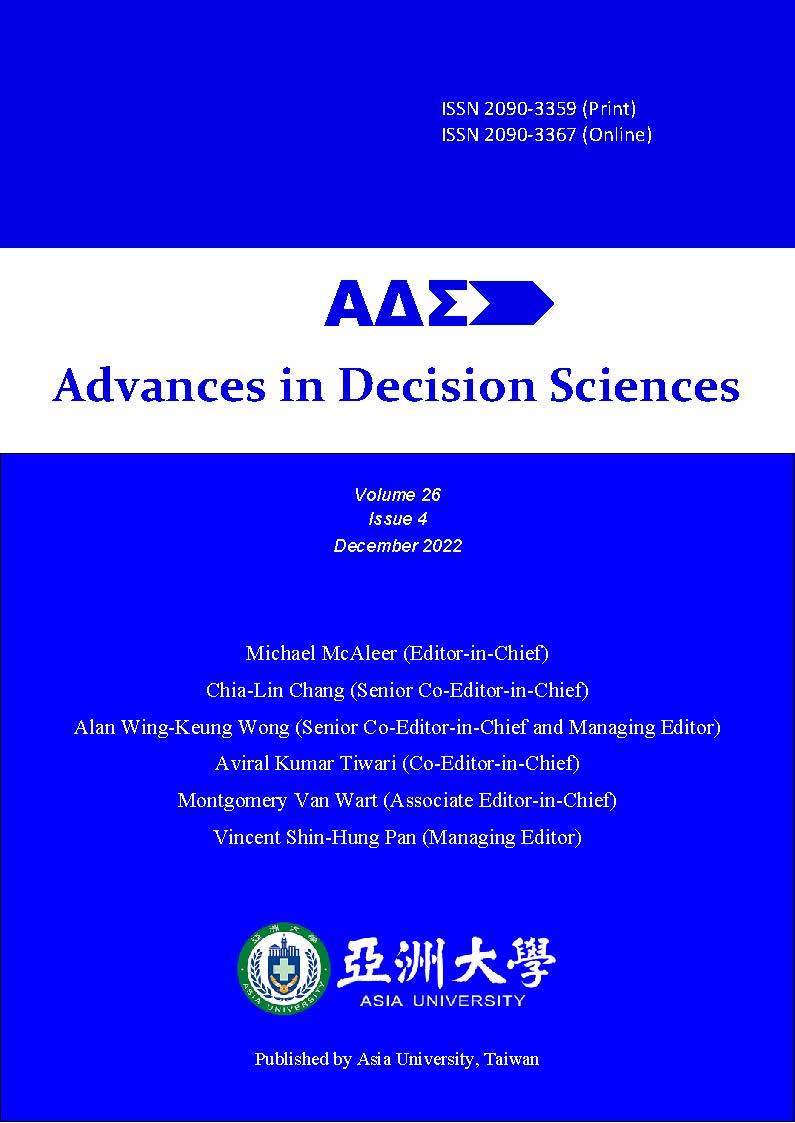
Published
Issue
Section
License
Copyright (c) 2022 Advances in Decision Sciences

This work is licensed under a Creative Commons Attribution-NonCommercial 4.0 International License.