Empirical Significance of Movements in Stock Trading Platforms in NSE Market Structure
DOI:
https://doi.org/10.47654/v26y2022i3p99-122Keywords:
Volume of Trade, Returns, Volatility, Trading Platforms, Conditional Volatility, GARCHAbstract
Information is the game-changer in the stock market environment. The distinction in terms of access to trade information (colocation, high frequency, direct market access, and smart order routing trades), machine interfered decisions making (Algorithmic-trades), and conventional trades (non-algorithmic trades) with or without mobile/internet connectivity defines market microstructure in a new perspective. The study evaluates movements along with price and volatility to understand the significance of trading movements in each platform. The study provides evidence that non-algorithmic trades are independent of market return and volatility while colocation trades are asymmetric. The GARCH framework identifies that colocation, internet, and algorithmic platforms explain volatility persistence for the study period. The study concludes that the trade movement of a specific platform acts as a proxy for information flow to identify signals for trade decisions by the traders of other platforms.
References
Andersen, T. G. (1996). Return volatility and trading volume: An information flow interpretation of stochastic volatility. The Journal of Finance, 51(1), 169-204. https://doi.org/10.1111/j.1540-6261.1996.tb05206.x
Kudryavtsev, A. (2020). Immediate and longer-term stock price dynamics following large stock price changes. Annals of Financial Economics, 15(01), 2050002. https://doi.org/10.1142/S2010495220500025
Balcilar, M., Bouri, E., Gupta, R., & Roubaud, D. (2017). Can volume predict Bitcoin returns and volatility? A quantiles-based approach. Economic Modelling, 64, 74-81. https://doi.org/10.1016/j.econmod.2017.03.019
Belhaj, F., & Abaoub, E. (2015). A Generalized Autoregressive Conditional heteroskedasticity Examination of the Relationship between Trading Volume and Conditional Volatility in the Tunisian Stock Market: Evidence for the Information Flow Paradigm. International Journal of Economics and Financial Issues, 5(2), 354-364.https://doi.org/10.1016/j.econmod.2017.03.019
Bekaert, G., & Wu, G. (2000). Asymmetric volatility and risk in equity markets. The review of financial studies, 13(1), 1-42. https://doi.org/10.1093/rfs/13.1.1
Bessembinder, H., Chan, K., & Seguin, P. J. (1996). An empirical examination of information, differences of opinion, and trading activity. Journal of Financial Economics, 40(1), 105-134. https://doi.org/10.1016/0304-405X(95)00839-7
Bouras, C., Christou, C., Gupta, R., & Suleman, T. (2019). Geopolitical risks, returns, and volatility in emerging stock markets: evidence from a panel GARCH model. Emerging Markets Finance and Trade, 55(8), 1841-1856. https://doi.org/10.1080/1540496X.2018.1507906
Brailsford, T J. (1996). The empirical relationship between trading volume, returns, and volatility. Accounting & Finance, 36(1), 89-111. https://doi.org/10.1111/j.1467-629X.1996.tb00300.x
Brogaard, J., Hagströmer, B., Nordén, L., & Riordan, R. (2015). Trading fast and slow: Colocation and liquidity. The Review of Financial Studies, 28(12), 3407-3443. https://doi.org/10.1093/rfs/hhv045
Chandra, A. (2012). Cause and effect between FII trading behavior and stock market return: The Indian experience. Journal of Indian Business Research. https://doi.org/10.1108/17554191211274794
Chen, G. M., Firth, M., & Rui, O. M. (2001). The dynamic relation between stock returns, trading volume, and volatility. Financial Review, 36(3), 153-174. https://doi.org/10.1111/j.1540-6288.2001.tb00024.x
Copeland, T. E. (1976). A Model for Asset Trading under the Assumption of Sequential Information Arrival. Journal of Finance, 31, 1149-1168. https://doi.org/10.2307/2326280
Engle, R. F., & Ng, V. K. (1993). Measuring and testing the impact of news on volatility. The journal of finance, 48(5), 1749-1778. https://doi.org/10.1111/j.1540-6261.1993.tb05127.x
Epps, T. W., & Epps, M. L. (1976). The stochastic dependence of security price changes and transaction volumes: Implications for the mixture-of-distributions hypothesis. Econometrica: Journal of the Econometric Society, 305-321. https://doi.org/10.2307/1912726
Ferreira, M. A., Matos, P., Pereira, J. P., & Pires, P. (2017). Do locals know better? A comparison of the performance of local and foreign institutional investors. Journal of Banking & Finance, 82, 151-164. https://doi.org/10.1016/j.jbankfin.2017.06.002
Fleming, J., Kirby, C., & Ostdiek, B. (2006). Stochastic volatility, trading volume, and the daily flow of information. The Journal of Business, 79(3), 1551-1590. https://doi.org/10.1086/500685
Frino, A., Prodromou, T., Wang, G. H., Westerholm, P. J., & Zheng, H. (2017). An empirical analysis of algorithmic trading around earnings announcements. Pacific-Basin Finance Journal, 45, 34-51. https://doi.org/10.1016/j.pacfin.2016.05.008
Graczyk, M. B., & Queiros, S. M. D. (2018). Volatility–Trading volume intraday correlation profiles and its nonstationary features. Physica A: Statistical Mechanics and its Applications, 508, 28-34. https://doi.org/10.1016/j.physa.2018.05.066
Gupta, S., Das, D., Hasim, H., & Tiwari, A. K. (2018). The dynamic relationship between stock returns and trading volume revisited: A MODWT-VAR approach. Finance Research Letters, 27, 91-98. https://doi.org/10.1016/j.frl.2018.02.018
Gupta, R., Lau, C. K. M., & Yoon, S. M. (2019). OPEC News Announcement Effect on Volatility in the Crude Oil Market: A Reconsideration, Advances in Decision Sciences, 23(4), 1-23.
Hendershott, T., & Riordan, R. (2009). Algorithmic trading and information. Manuscript, University of California, Berkeley. DOI: https://doi.org/10.1017/S0022109013000471
Ilomäki, J., & Laurila, H. (2018). The noise trader effect in a Walrasian financial market. Advances in Decision Sciences, 22, 1-14. https://doi.org/10.47654/v22y2018i1p405-419
Jain, A., & Biswal, P. C. (2022). FII Trading Pressure and Stock Volatility in India. In Studies in International Economics and Finance (pp. 633-646). Springer, Singapore. DOI: 10.1007/978-981-16-7062-6_32
Kao, Y. S., Chuang, H. L., & Ku, Y. C. (2020). The empirical linkages among market returns, return volatility, and trading volume: Evidence from the S&P 500 VIX Futures. The North American Journal of Economics and Finance, 54, 100871. https://doi.org/10.1016/j.najef.2018.10.019
Kapusuzoglu, A., & Ceylan, N. B. (2018). Trading volume, volatility, and GARCH effects in Borsa Istanbul. In Strategic design and innovative thinking in business operations (pp. 333-347). Springer, Cham. https://doi.org/10.1007/978-3-319-77622-4_17
Karpoff, J. M. (1987). The relation between price changes and trading volume: A survey. Journal of Financial and Quantitative Analysis, 22(1), 109-126. https://doi.org/10.2307/2330874
Kim, N., Lučivjanská, K., Molnár, P., & Villa, R. (2019). Google searches and stock market activity: Evidence from Norway. Finance Research Letters, 28, 208-220. https://doi.org/10.1016/j.frl.2018.05.003
Koubaa, Y., & Slim, S. (2019). The relationship between trading activity and stock market volatility: Does the volume threshold matter? Economic Modelling, 82, 168-184. https://doi.org/10.1016/j.econmod.2019.01.003
Kumar, S. (2019). The relationship between trading volume and exchange rate volatility: linear or nonlinear? International Journal of Managerial Finance. https://doi.org/10.1108/IJMF-02-2018-0060
Lakonishok, J., & Maberly, E. (1990). The weekend effect: Trading patterns of individual and institutional investors. The Journal of Finance, 45(1), 231-243. https://doi.org/10.1111/j.1540-6261.1990.tb05089.x
Lang, Q., Wang, J., Ma, F., Huang, D., & Ismail, M. W. M. (2021). Is the Baidu index really powerful to predict the Chinese stock market volatility? New evidence from the internet information. China Finance Review International. https://doi.org/10.1108/CFRI-03-2021-0047
Lamoureux, C. G., & Lastrapes, W. D. (1990). Heteroskedasticity in stock return data: volume versus GARCH effects. The journal of finance, 45(1), 221-229. https://doi.org/10.1111/j.1540-6261.1990.tb05088.x
Lakshmi, P. (2012). FII Trading Volume and Symmetric Volatility: Analysis from Indian Spot Market. Vilakshan: The XIMB Journal of Management, 9(1).
Lakshmi, V. D. M. V. (2022). Do Exchange Traded Funds in India Have Tracking and Pricing Efficiency? Advances in Decision Sciences, 26(2), 1-25. https://doi.org/10.47654/v26y2022i2p1-25
Lee, C. M., & Swaminathan, B. (2000). Price momentum and trading volume. the Journal of Finance, 55(5), 2017-2069. https://doi.org/10.1111/0022-1082.00280
Li, J., & Wu, C. (2006). Daily return volatility, bid‐ask spreads, and information flow: Analyzing the information content of the volume. The Journal of Business, 79(5), 2697-2739. https://doi.org/10.1086/505249
Liu, M., Lee, C. C., & Choo, W. C. (2021). An empirical study on the role of trading volume and data frequency in volatility forecasting. Journal of Forecasting, 40(5), 792-816. https://doi.org/10.1002/for.2739
Mahajan, S., & Singh, B. (2008). An empirical analysis of stock price-volume relationship in the Indian stock market. Vision, 12(3), 1-13. https://doi.org/10.1177/097226290801200301
Mahajan, S., & Singh, B. (2009). The empirical investigation of the relationship between return, volume, and volatility dynamics in the Indian stock market. Eurasian Journal of Business and Economics, 2(4), 113-137. https://www.ejbe.org/index.php/EJBE/article/view/27
Moslehpour, M., Al-Fadly, A., Ehsanullah, S., Chong, K. W., Xuyen, N. T. M., & Tan, L. P. (2022). Assessing financial risk spillover and panic impact of COVID-19 on European and Vietnam stock markets. Environmental Science and Pollution Research, 29(19), 28226-28240. https://doi.org/10.1007/s11356-021-18170-2
Naufa, A. M., Lantara, I. W. N., & Lau, W. Y. (2019). The impact of foreign ownership on return volatility, volume, and stock risks: Evidence from ASEAN countries. Economic Analysis and Policy, 64, 221-235. https://doi.org/10.1016/j.eap.2019.09.002
Omran, M. F., & McKenzie, E. (2000). Heteroscedasticity in stock returns data revisited: volume versus GARCH effects. Applied Financial Economics, 10(5), 553-560. https://doi.org/10.1080/096031000416433
Sampath, A., & Garg, P. (2019). Contemporaneous and Causal Relationship between Returns and Volumes: Evidence from Nifty Futures. International Review of Finance, 19(3), 653-664. https://doi.org/10.1111/irfi.12175
Schuppli, M., & Bohl, M. T. (2010). Do foreign institutional investors destabilize China’s A-share markets? Journal of International Financial Markets, Institutions, and Money, 20(1), 36-50. https://doi.org/10.1016/j.intfin.2009.10.004
Sharma, J. L., Mougoue, M., & Kamath, R. (1996). Heteroscedasticity in stock market indicator return data: volume versus GARCH effects. Applied Financial Economics, 6(4), 337-342. https://doi.org/10.1080/096031096334132
Shen, D., Li, X., & Zhang, W. (2018). Baidu news information flow and return volatility: Evidence for the Sequential Information Arrival Hypothesis. Economic Modelling, 69, 127-133. https://doi.org/10.1016/j.econmod.2017.09.012
Smirlock, M., & Starks, L. (1985). A further examination of stock price changes and transaction volume. Journal of Financial Research, 8(3), 217-226. https://doi.org/10.1111/j.1475-6803.1985.tb00404.x
Smirlock, M., & Starks, L. (1988). An empirical analysis of the stock price-volume relationship. Journal of Banking & Finance, 12(1), 31-41. https://doi.org/10.1016/0378-4266(88)90048-9
Srilakshminarayana, G. (2021). Tail Behaviour of the Nifty-50 Stocks during Crises Periods. Advances in Decision Sciences, 25(4), 1-36. https://doi.org/10.47654/v25y2021i4p115-151
TAJMAZINANI, M., HASSANI, H., RAEI, R., & ROUHANI, S. (2022). Modeling stock price movement prediction based on news sentiment analysis and deep learning. Annals of Financial Economics, 17(01), 2250003. https://doi.org/10.1142/S2010495222500038
Yuan, P. (2019). Forecasting realized volatility dynamically based on adjusted dynamic model averaging (AMDA) approach: evidence from China’s stock market. Annals of Financial Economics, 14(04), 1950022. https://doi.org/10.1142/S2010495219500222
Zhang, Y., Feng, L., Jin, X., Shen, D., Xiong, X., & Zhang, W. (2014). Internet information arrival and volatility of SME PRICE INDEX. Physica A: Statistical Mechanics and its Applications, 399, 70-74. https://doi.org/10.1016/j.physa.2013.12.034
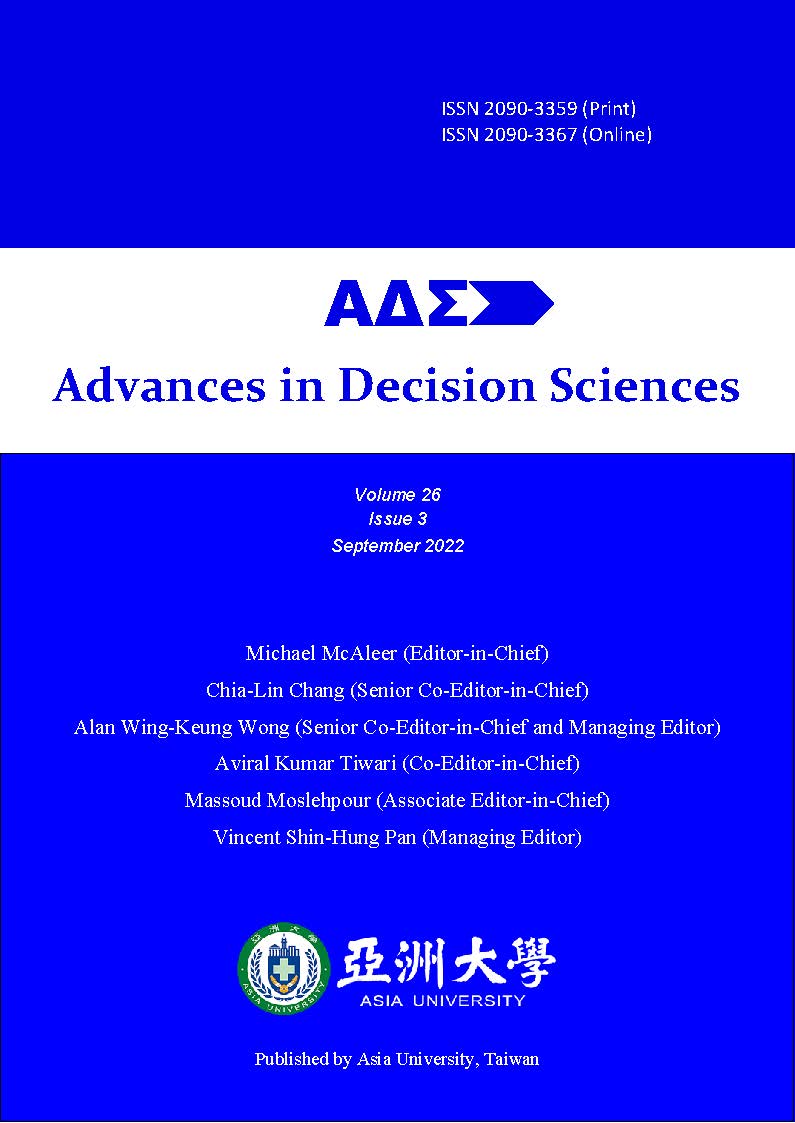
Published
Issue
Section
License
Copyright (c) 2022 Advances in Decision Sciences

This work is licensed under a Creative Commons Attribution-NonCommercial 4.0 International License.